tech news, Artificial Intelligence
Making Gen AI Sustainable
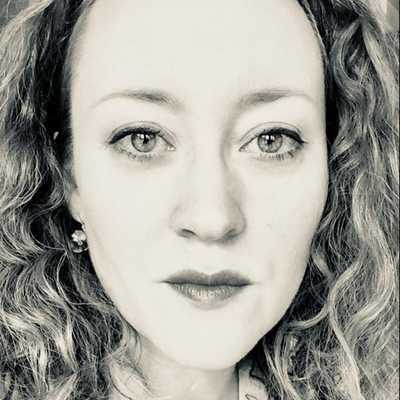
By Laura Cowan
Laura K. Cowan is a tech editor and journalist whose work has focused on promoting sustainability initiatives for automotive, green tech, and conscious living media outlets.
Gen AI Has a Sustainability Problem
"GenAI," or generative artificial intelligence, has a sustainability problem. While businesses are using the new technology to train data sets and extract patterns for use for everything from content generation to legal language compliance, the training of one AI model can use as much as 100 homes worth of electricity in one year.
GenAI can be made more sustainable by considering various aspects of its development, deployment, and use. What does that involve? Sustainability in AI includes minimizing environmental impact, remembering ethical concerns, and planning for long-term societal well-being.
Here are some ways in which GenAI can become more sustainable:
- Energy Efficiency: AI models, particularly large ones like GPT-3, require significant computational power, which in turn demands a lot of energy. Sustainable GenAI should focus on developing energy-efficient models and optimizing the hardware used for training and deployment.
- Green Computing: Utilize renewable energy sources and energy-efficient data centers for training and running AI models. Using green computing practices reduces the carbon footprint of AI applications.
- Reducing Data Center Emissions: Minimize the emissions associated with data centers by locating them in areas with access to renewable energy sources and implementing cooling technologies that reduce energy consumption. This also means not competing with other uses of energy, which is a frequent complaint in the small towns and out-of-the-way locations where servers for AI are often located, eating up massive amounts of local energy resources and putting out sound pollution at a minimum, emissions at worst.
- Model Efficiency: Develop and promote smaller, more efficient AI models that can perform tasks with fewer parameters. This reduces the computational resources required for training and inference. Knowing how to scale your use of AI can go a long way toward solving this concern. The same way we manage cloud budgets we will soon be managing AI resources.
- Ethical AI: Ensure that GenAI models are developed and used in ways that align with ethical guidelines, respecting human rights, privacy, and inclusivity. A sustainable AI respects individual privacy and data protection laws.
- Bias Mitigation: Address bias and fairness issues in AI systems to ensure they do not perpetuate discrimination or inequality. Developing AI systems that are unbiased and fair is crucial for long-term societal sustainability.
- Transparency and Accountability: Encourage transparency in AI decision-making processes and create mechanisms for accountability. This fosters trust and ensures responsible AI use.
- Extended Product Lifecycles: Extend the useful life of AI models by providing updates and support, reducing the need for frequent model replacements and, consequently, reducing electronic waste.
- Data Minimization: Collect and store only the necessary data to train and operate GenAI systems. Reducing data usage limits the risk of privacy breaches and data exposure. Expect this to be a hot topic for the next few years as privacy and data collection involved in AI training models is fought out in the courts.
- Regulation and Governance: Support regulatory frameworks and standards that guide the responsible development and use of AI. Government policies and industry standards can ensure that AI aligns with sustainable practices.
- Education and Awareness: Promote education and awareness about the environmental and ethical aspects of AI among developers, organizations, and the general public.
- Collaboration: Foster collaboration between governments, organizations, and researchers to work on AI sustainability. Encourage information sharing, research, and development to address sustainability challenges.
- Recycling and E-Waste Management: Implement responsible recycling and e-waste management practices for AI hardware components to reduce environmental impact.
- Local AI Processing: Whenever possible, opt for local AI processing on edge devices rather than cloud-based solutions. This reduces latency and energy consumption.
- Carbon Offsetting: Consider carbon offsetting initiatives to counterbalance the emissions associated with AI development and use. Make sure if you go this route you find reputable third-party certified programs for carbon offsets, as some companies sell questionable junk credits that don't offset much.
Sustainability in AI requires a holistic approach that balances the benefits of AI with its environmental and societal impacts, and this will take a while with all brains on deck to balance out. Sustainability in AI involves continuous improvement, awareness, and responsible practices throughout the entire AI lifecycle, from development to deployment and beyond.
What are your plans for GenAI, and do you have a way to make it sustainable to match your company's emissions and sustainability goals?
Need help? Cronicle is currently signing up participants in a survey to learn what businesses need when it comes to revamping and publicizing their sustainbility goals through content marketing. To learn more, please email the editor at laura [at] cronicle.press for more information.
tech news, AI, gen AI, sustainability, climate